<!-- poster arab-american slides: 1,21-23,25-37,56 1-3,20-37,56-57 spatial statistics disease environment --> <style> .pull-left-50 { float: left; width: 50%; } .pull-right-50 { float: right; width: 50%; } .pull-left-60 { float: left; width: 60%; } .pull-right-40 { float: right; width: 40%; } .pull-right-40-padding { float: right; width: 38%; padding-left: 10px } </style> <style> .pull-left-70 { float: left; width: 70%; } .pull-right-30 { float: right; width: 30%; } </style> <div class = "content"> <br> <center> <div style = 'margin-top: -60px; margin-bottom: -100px; margin-left:-30px; margin-right:-30px;'> <p class="text-center" style = 'font-size: 56px; line-height:1.5; font-weight:bold;'>Geospatial Data Science for Public Health Surveillance</p> <!-- <p class="text-center" style = 'font-size: 56px; line-height:1.5; font-weight:bold;'>Bayesian risk models for tropical disease mapping</p> <p class="text-center" style = 'font-size: 48px; line-height:1.5; font-weight:bold;'>How geostatistics can help with decision-making in global health</p> <p class="text-center" style = 'font-size: 38px; font-weight:bold; margin-top:-40px;'>Case studies in tropical disease mapping</p><br> --> </div> </center> <br> <table style="margin:0px; margin-left:-10px; border-top:0; border-bottom:0;"> <tr> <td style="width: 440px;"> <div style = 'padding: 40px; padding-left: 40px; font-size: 32px; font-weight:bold; margin-top: 10px; margin-bottom: -50px;'> <br> Paula Moraga, Ph.D.<br> </div> <div style = 'padding: 40px; padding-left: 40px; font-size: 28px; margin-bottom: -60px;'> Asst. Professor of Statistics </div> <div style = 'padding: 40px; padding-left: 40px; font-size: 28px; margin-bottom: -55px;'> King Abdullah University of Science and Technology (KAUST), Saudi Arabia </div> <div style = 'padding: 40px; padding-left: 40px; font-size: 26px; line-height:1.5;margin-bottom: 40px;'> <a href='http://twitter.com/Paula_Moraga_' target='_blank'> <i class='fa fa-twitter fa-fw'></i> @Paula_Moraga_</a><br> <a href='https://Paula-Moraga.github.io/' target='_blank'> <i class='fa fa-globe fa-fw'></i> www.PaulaMoraga.com</a><br> <!-- <a href='http://bit.ly/prestdm' target='_blank'><i class='fa fa-link fa-fw'></i> www.paulamoraga.com/presentation-geohealth/</a><br> --> </div> </td> <td> <center> <img src="./figures/logogeohealth.png" height = "200" alt = "a png"><br><br> <img src="./figures/Statistics at KAUST_Logo for digital use_small.png" height = "160" alt = "a png"> </center> </td> </tr> </table> </div> <!-- #--- .pull-left-70[ # Education <p style = 'margin-bottom: -10px;'></p> - **PhD Mathematics**, University of Valencia, 2012 #-- Comment (Extraordinary Doctoral Award) -- - **MSc Biostatistics**, Harvard University, 2011 - **BSc Mathematics**, University of Valencia, 2006 Erasmus Johannes Gutenberg University Mainz <p style = 'margin-bottom: 50px;'></p> ] .pull-right-30[ <img src="./figures/valencialogo.png" style="width:100%;"/> <img src="./figures/harvardlogo.png" style="width:100%;"/> ] # Experience <img src="./figures/jobstimeline.png" style="width:100%;"/> --> <!-- #--- **BSc Mathematics**, University of Valencia, Johannes Gutenberg U. Mainz #-- Openfinance #-- Start PhD and collaboration with cancer registry #-- **MSc Biostatistics**, Harvard University #-- Harvard Medical School, Brigham and Women's Hospital, MUSC <br> Google Summer of Code <br> European Centre for Disease Prevention and Control (ECDC) #-- **PhD Mathematics**, University of Valencia #-- Lancaster University <br> Harvard University <br> London School of Hygiene and Tropical & Medicine <br> Queensland University of Technology (QUT) <br> Lancaster University <br> University of Bath <br> #-- **KAUST** Prof. Statistics and PI GeoHealth <img src="./figures/Statistics at KAUST_Logo for digital use_small.png" height = "100" alt = "a png"> <img src="./figures/logogeohealth.png" height = "100" alt = "a png"> --> <!-- #--- <div style="margin-top:-20px"></div> # Who am I? <div style="margin-top:-20px"></div> <style type="text/css"> .circular--square { border-radius: 50%; } </style> <table style="margin:20px; border-top:0; border-bottom:0; margin-bottom: -20px;"> <tr> <td> <p style="font-size:25px"><b>Paula Moraga, Ph.D.</b></p> Assistant Professor of Statistics <br>for Public Health at KAUST<br><br> PI Geospatial Statistics and Health Surveillance Research Group<br><br> <img src="./figures/Statistics at KAUST_Logo for digital use_small.png" height = "120" alt = "a png"> <img src="./figures/logogeohealth.png" height = "120" alt = "a png"> </td> <td style="width:40%"> <img class = "circular--square" src="./figures/paula.png" width = "200" alt = "a png"><br><br> <a href='http://twitter.com/Paula_Moraga_' target='_blank'><i class='fa fa-twitter fa-fw'></i> @Paula_Moraga_</a><br> <a href='https://Paula-Moraga.github.io/' target='_blank'><i class='fa fa-globe fa-fw'></i> www.PaulaMoraga.com</a><br> </td> </tr> </table> <br> <i class='fa fa-map-marked-alt fa-fw'></i> Geospatial data analysis, statistical modeling<br> <i class='fa fa-hospital fa-fw'></i> Spatial epidemiology, disease mapping, health surveillance<br> <i class='fa fa-laptop fa-fw'></i> Development of R packages and interactive visualization applications<br> <i class='fa fa-book fa-fw'></i> Author book Geospatial Health Data http://bit.ly/bookgeo<br> <i class='fa fa-graduation-cap fa-fw'></i> PhD Mathematics, Valencia. Master's Biostatistics, Harvard<br> --> --- background-image: url(./figures/overview.png) background-size: contain <!-- #--- <div style="margin-top:-40px"></div> ## https://www.paulamoraga.com/book-geospatial/ <div style="margin-top:-10px"></div> .pull-left[ <center> <img src="./figures/bookcover.jpg" style="margin-top:-5px; margin-left:-10px; width:100%;"/> </center> ] .pull-right[ <div style="margin-top:-33px"></div> <blockquote class="twitter-tweet" data-lang="en"><p lang="en" dir="ltr"> <a href="https://twitter.com/Paula_Moraga_/status/1136345687942152194"></a></blockquote> <script async src="https://platform.twitter.com/widgets.js" charset="utf-8"></script> ] --> --- <div style="margin-top:-40px"></div> <!-- https://annakrystalli.me/literate-programming/ --> # Books <div style="margin-top:-10px"></div> .pull-left[ <center> <img src="./figures/bookcover.jpg" style="margin-top:-5px; margin-left:-10px; width:100%;"/> </center> <small><small> <a href="https://www.paulamoraga.com/book-geospatial/">https://www.paulamoraga.com/book-geospatial/</a> </small></small> ] .pull-left[ <center> <img src="./figures/bookcoverspatial.jpg" style="margin-top:-5px; margin-left:10px; width:100%;"/> </center> <small><small> <a href="https://www.paulamoraga.com/book-spatial/"> https://www.paulamoraga.com/book-spatial/</a> </small></small> ] --- <div style="margin-top:-20px"></div> <h3>Geospatial Health Data: Modeling and Visualization with R-INLA and Shiny (2019) <a href="https://www.paulamoraga.com/book-geospatial/"> http://www.paulamoraga.com/book-geospatial/ </a> </h3> <div style="margin-top:-10px"></div> <center> <img src="./figures/bookcover.jpg" style="margin-top:-5px; margin-left:-10px; width:30%;"/> <img src="./figures/bookcoverchinese.jpg" style="margin-top:-5px; margin-left:-10px; width:37%;"/> </center> <div style="margin-top:-10px"></div> - Manipulate and transform point, areal, raster data, create maps with R - Fit and interpret Bayesian spatial, spatio-temporal models w/ INLA, SPDE - Interactive viz, reproducible reports, dashboards, and Shiny apps - Health examples (model disease risk and quantify risk factors in different settings) but methods also useful to analyze geospatial data in other fields <!-- https://xuanshu.hep.com.cn/front/book/findBookDetails?bookId=64a05ce2938b7cc2960f0603 --> <!-- - Manipulate and transform point, areal and raster data,<br> and create maps with R - Fit and interpret Bayesian spatial and spatio-temporal models with INLA and SPDE - Interactive visualizations, reproducible reports, dashboards, and Shiny apps - Model disease risk and quantify risk factors in different settings - Health examples but methods useful to analyze georeferenced data in other fields such as ecology or criminology --> --- <div style="margin-top:-20px"></div> <h3>Spatial Statistics for Data Science: Theory and Practice with R (2023) <a href="https://www.paulamoraga.com/book-spatial/"> http://www.paulamoraga.com/book-spatial/ </a> </h3> .pull-left[ <div style="margin-top:-20px"></div> - Statistical methods, modeling approaches, and visualizations to analyze spatial data using R - **Spatial data**: types, retrieval, manipulation and visualization - **Areal data**: spatial neighborhood matrices, autocorrelation, spatial models - **Geostatistical data**: spatial interpolation methods, kriging, model-based geostatistics - **Point patterns**: intensity estimation, clustering, LGCPs - Reproducible examples in disease risk, air pollution, species distribution, crime mapping, real state analyses ] .pull-right[ <center> <img src="./figures/bookcoverspatial.jpg" style="margin-top:0px; margin-left:-10px; width:95%;"/> </center> ] <!-- https://annakrystalli.me/literate-programming/ --> <!-- #--- class: inverse # Ciencia de datos geoespaciales para la vigilancia de la salud pública <br> ## Datos y métodos geoespaciales ## Aplicaciones en enfermedades tropicales ## Software estadístico ## Investigación actual --> --- class: inverse # Geospatial Data Science for<br> Public Health Surveillance ## Geospatial data and methods ## Spatial methods ## Spatial epidemiology studies ## Statistical software ## Current research <!-- Now have commented point patterns --> --- class: inverse, center, middle # Geospatial data and methods <!-- for<br> disease surveillance --> <!-- #--- <img src="figures/snow-cholera-map.jpg" width="90%" style="display: block; margin: auto;" /> --> --- background-image: url(figures/snow-cholera-map-pump-top-margin.png) background-size: contain <div style="margin-top:-20px"></div> # John Snow's map of cholera, London, 1854 <!-- #--- ## Geospatial modeling and visualization with R - Methods to analyze geospatial health data that enable to quantify disease burden, understand geographic and temporal patterns, identify risk factors, and measure inequalities <img src="./figures/typesspatialdata.png" style="width:100%;"/> - Maps and other visualizations that enable to represent disease risk and risk factors, and presentation options such as interactive dashboards to communicate results - Examples focus on geospatial health data but the methods are also useful in others fields that use georeferenced data including epidemiology, ecology, demography and criminology --> --- # Geospatial methods for disease surveillance Geospatial methods use data on disease cases, individuals at risk, and risk factors such as demographic and environmental factors to - Understand geographic and temporal patterns - Highlight areas of high risk and detect clusters - Identify potential risk factors - Measure inequalities - Quantify the excess of disease risk close to a putative source - Early detection of outbreaks 🗣 Maps and other visualizations enable to represent disease risk and risk factors, and interactive dashboards facilitate the communication of results ✍️ Results guide decision makers to better allocate limited resources and to design strategies for disease prevention and control 🌍 Many of these methods are also useful to analyze georeferenced data in others fields such as ecology, environment and criminology <!-- **Data**: Methods use information about disease cases, individuals at risk, and potential risk factors such as demographic and environmental factors **Disease models** describe the variability in disease risk as a function of explanatory variables and random effects to account for unexplained variability. Models allow straightforward extensions to estimate covariate effects, and handle spatio-temporal data and multiple diseases --> --- background-image: url(figures/arealgeostatisticalpointpatterns.png) background-size: contain background-position: left 50% <div style="margin-bottom:-20px"></div> # Types of spatial data <div style="margin-bottom:500px"></div> [Moraga and Lawson, Computational Statistics & Data Analysis, 2012](https://doi.org/10.1016/j.csda.2011.11.011) [Moraga et al., Parasites & Vectors, 2015](https://doi.org/10.1186/s13071-015-1166-x) [Moraga and Montes, Statistics in Medicine, 2011](https://doi.org/10.1002/sim.4160) --- <div style="margin-top:-40px"></div> # Areal data <div style="margin-top:-40px"></div> <img src="./figures/georgiaLBW.png" width="40%" style="display: block; margin: auto;" /> <div style="margin-top:-10px"></div> **Standardized Mortality Ratio (SMR)** is often used to estimate disease risk <div style="margin-top:-10px"></div> `$$SMR \mbox{ in area } i = SMR_i = \frac{Y_i}{E_i} = \frac{\mbox{number observed cases in area } i}{\mbox{number expected cases in area } i}$$` `\(SMR_i = 1\)` `\(\rightarrow\)` same number of cases observed as expected `\(SMR_i > 1\)` `\(\rightarrow\)` more number of cases observed than expected (high risk) `\(SMR_i < 1\)` `\(\rightarrow\)` less number of cases observed than expected (low risk) **Limitations** <div style="margin-top:-10px"></div> SMRs may be misleading and unreliable in areas with small populations or rare diseases. Models enable to incorporate covariates and borrow information from neighboring areas to obtain smoothed relative risks --- <div style="margin-top:-40px"></div> # Areal models Model to estimate disease relative risk `\(\theta_i\)` in areas `\(i=1,\ldots,n\)` `$$Y_i|\theta_i \sim Poisson(E_i \times \theta_i)$$` `$$\log(\theta_i) = \boldsymbol{z}_i \boldsymbol{\beta} + u_i + v_i$$` - `\(Y_i\)`: number observed cases in area `\(i\)` - `\(E_i\)`: number expected cases in area `\(i\)` - `\(\theta_i\)`: relative risk in area `\(i\)` Fixed effects quantify the effects of the covariates on the disease risk - `\(\boldsymbol{z}_i = (1, z_{i1}, \ldots, z_{ip})\)` vector of the intercept and covariates `\(\beta = (\beta_0, \beta_1, \ldots, \beta_p)'\)` coefficient vector Random effects represent residual variation which is not explained by the available covariates - `\(u_i\)`: structured spatial effect to account for the spatial dependence between relative risks (areas that are close show more similar risk than areas that are not close) - `\(v_i\)`: unstructured spatial effect to account for independent noise --- # Spatial neighborhood matrix ```r library(spdep) nb <- poly2nb(map) ``` ```r ## [[1]] ## [1] 21 28 67 ## ## [[2]] ## [1] 3 4 10 63 65 ``` <center> <img src="./figures/RCode/mapneighbors.png" style="width:55%;"/> </center> --- # Inference using INLA `$$Y_i|\theta_i \sim Poisson(E_i \times \theta_i)$$` `$$\log(\theta_i) = \boldsymbol{z}_i \boldsymbol{\beta} + u_i + v_i$$` ```r library(INLA) formula <- Y ~ cov + f(idareau, model = "besag", graph = g, scale.model = TRUE) + f(idareav, model = "iid") res <- inla(formula, family = "poisson", data = map@data, E = E, control.predictor = list(compute = TRUE)) # Posterior mean and 95% CI map$RR <- res$summary.fitted.values[, "mean"] map$LL <- res$summary.fitted.values[, "0.025quant"] map$UL <- res$summary.fitted.values[, "0.975quant"] ``` R-INLA: http://www.r-inla.org/ --- # Visualization ```r library(leaflet) pal <- colorNumeric(palette = "YlOrRd", domain = map$SIR) leaflet(map) %>% addTiles() %>% addPolygons(color = "grey", weight = 1, fillColor = ~ pal(SIR)) %>% addLegend(pal = pal, values = ~SIR, title = "SIR") ``` <center> <img src="./figures/RCode/m.gif" style="width:50%;"/> </center> --- <div style="margin-top:-10px"></div> # Geostatistical data <center> <div style="margin-left:-70px; margin-right:-20px; width:120%;"> <img src="./figures/mbg.png" style="width:100%;"/> </div> </center> --- <div style="margin-top: -45px;"> # Geostatistical models <div style="margin-top: -20px;"> Models to predict prevalence at unsampled locations `$$Y_i|P(\boldsymbol{x}_i)\sim \mbox{Binomial} (n_i, P(\boldsymbol{x}_i))$$` `$$\mbox{logit}(P(\boldsymbol{x}_i)) = \boldsymbol{z}_i \boldsymbol{\beta} + S(\boldsymbol{x}_i) + u_i$$` `\(Y_i\)` number people positive, `\(n_i\)` number people tested, `\(P(x_i)\)` prevalence at `\(\boldsymbol{x}_i\)` <div style="margin-top: -10px;"> Covariates based on characteristics known to affect disease transmission (temperature, precipitation, vegetation, elevation, population density, etc.) <div style="margin-top: -10px;"> <!-- (temperature, precipitation, vegetation, elevation, distance to water bodies, urbanization, land cover, population density, etc) Random effects (Gaussian process with Matern covariate funcion, Independent risk) --> Random effects model residual variation not explained by covariates <center> <img src="./figures/covgrf.png" style="width:80%;"/> </center> --- # Triangulated mesh for SPDE approach ```r library(INLA) mesh <- inla.mesh.2d( loc = coo, offset = c(50, 100), cutoff = 1, max.edge = c(30, 60)) ``` <center> <img src="./figures/RCode/mesh.png" style="width:70%;"/> </center> --- # Spatial covariates ```r library(raster) r <- getData(name = "alt", country = "GMB", mask = TRUE) ``` <center> <img src="./figures/RCode/altitude.png" style="width:70%;"/> </center> --- # Inference using INLA and SPDE `$$Y_i|P(\boldsymbol{x}_i)\sim \mbox{Binomial} (n_i, P(\boldsymbol{x}_i))$$` `$$\mbox{logit}(P(\boldsymbol{x}_i)) = \boldsymbol{z}_i \boldsymbol{\beta} + S(\boldsymbol{x}_i) + u_i$$` ```r library(INLA) formula <- y ~ 0 + b0 + alt + f(s, model=spde) + f(idu, model="iid") res <- inla(formula, family = "binomial", Ntrials = numtrials, control.family = list(link = "logit"), data = inla.stack.data(stk.full), control.predictor = list(compute = TRUE, link = 1, A = inla.stack.A(stk.full))) index <- inla.stack.index(stack = stk.full, tag = "pred")$data # Posterior mean and 95% CI prev_mean <- res$summary.fitted.values[index, "mean"] prev_ll <- res$summary.fitted.values[index, "0.025quant"] prev_ul <- res$summary.fitted.values[index, "0.975quant"] ``` <!-- #--- <div style="margin-top:-40px"></div> # Point patterns <div style="margin-top:-100px"></div> <center> <div style="margin-left:70px; width:40%;"> <img src="./figures/pointdataKidney.png"/> </div> </center> <div style="margin-top:-20px"></div> Assume point pattern `\(\{s_i: i=1, \ldots, n\}\)` has been generated as a realization of a point process `\(Z = \{Z(s): s \in \mathbb{R}^2\}\)` Point processes are stochastic models that describe the locations of events of interest and possibly some additional information such as marks that inform about different types of events (e.g., cases and controls) A point process model can be used to identify patterns in the distribution of the observed locations, estimate the intensity of events, and learn about the correlation between the locations and spatial covariates #--- # Point process models **Homogeneous Poisson process** - Number of events in any region `\(A\)` distributed as `\(\mbox{Poisson}(\lambda |A|)\)`<br> where `\(\lambda\)` constant value denoting the intensity and `\(|A|\)` area of `\(A\)` - Number of events in disjoint regions are independent - Thus, an event is equally likely to occur at any location within the study region, regardless of the locations of other events **Log-Gaussian Cox process (LGCP)** - Poisson process with a varying intensity which is itself a stochastic process `\(\Lambda(s) = exp(Z(s))\)`, `\(Z = \{Z(s): s \in \mathbb{R}^2\}\)` Gaussian process - Conditional on `\(\Lambda(s)\)`, LGCP is a Poisson process with intensity `\(\Lambda(s)\)` - Thus, the number of events in any region `\(A\)` distributed as `\(\mbox{Poisson}(\int_A \Lambda(s)ds)\)` and the event locations are an independent random sample from the distribution on `\(A\)` with probability density proportional to `\(\Lambda(s)\)` #--- # Log-Gaussian Cox process Locations are a realization of a LGCP with varying intensity which is a stochastic process `\(\Lambda(s)= exp(\eta(s))\)`, `\(\eta = \{\eta(s): s \in \mathbb{R}^2\}\)` Gaussian process Fit model by approximating using a gridding approach: - Discretize study region into a grid `\(\{s_{ij}\}\)` with `\(n_1 \times n_2\)` cells - Mean number of events in cell `\(s_{ij}\)` is `\(\Lambda_{ij}=\int_{s_{ij}} exp(\eta(s))ds \approx |s_{ij}| exp(\eta_{ij})\)`, where `\(|s_{ij}|\)` is the cell area - Number of events in cell `\(s_{ij}\)` are independent and Poisson distributed `$$y_{ij}|\eta_{ij} \sim Poisson(|s_{ij}| exp(\eta_{ij}))$$` `$$\eta_{ij} = \beta_0 + \beta_1 \times cov(s_{ij}) + f_s(s_{ij}) + f_u(s_{ij})$$` #--- # Computational grid Discretize study region into a grid and count the number of points in each cell ```r library(rnaturalearth) library(raster) # Retrieve study region boundaries map <- ne_countries(type = "countries", country = country) # Create raster grid resolution <- 0.1 r <- raster(map, resolution = resolution) # Number points in each cell r[] <- 0 tab <- table(cellFromXY(r, dpts)) r[as.numeric(names(tab))] <- tab # Grid to be passed to INLA grid <- rasterToPolygons(r) ``` #--- # Inference using INLA `$$y_{ij}|\eta_{ij} \sim Poisson(|s_{ij}| exp(\eta_{ij}))$$` `$$\eta_{ij} = \beta_0 + \beta_1 \times cov(s_{ij}) + f_s(s_{ij}) + f_u(s_{ij})$$` ```r library(INLA) formula <- Y ~ 1 + cov + f(id, model = "rw2d", nrow = nrow, ncol = ncol) + f(id2, model = "iid") res <- inla(formula, family = "poisson", data = grid@data, E = cellarea, control.predictor = list(compute = TRUE)) # Posterior mean number of events and 95% CI grid$NE <- res$summary.fitted.values[, "mean"] * cellarea grid$LL <- res$summary.fitted.values[, "0.025quant"] * cellarea grid$UL <- res$summary.fitted.values[, "0.975quant"] * cellarea ``` --> --- class: inverse # Spatial methods for disease surveillance <div style="margin-top:80px;"> ## 1. Precision disease mapping ## 2. Velocities of disease spread ## 3. Clustering --- class: inverse, center, middle # Precision disease mapping --- .pull-left-50[ # Disease mapping Disease maps help decision-makers to target health interventions and directing resources where most needed Map shows malaria prevalence in Mozambique. Area-level data unable to show how disease risk varies within areas Areal estimates make difficult targeting health interventions and directing resources where most needed ] .pull-right-50[ <img src="./figures/mozambique1.png" style="width:150%;"/> ] [Moraga, et al., Spatial Statistics, 2017](https://doi.org/10.1016/j.spasta.2017.04.006) [Zhong and Moraga, JABES, 2023](https://doi.org/10.1007/s13253-023-00559-w) --- # Disaggregate area-level data High-resolution estimates permit to find differences in disease risk within study regions, and identify areas and groups of people at higher risk <img src="./figures/mozambique12precision2.png" style="width:100%;"/> --- background-image: url(./figures/regressionrescov.png) background-size: contain background-position: 0 250px # Spatially misaligned data Quantifying risk factors with misaligned data. Investigate relationship between a response variable and explanatory variables available at different spatial scales (e.g., lung cancer at county level and smoking at state level) <div style = "margin-top: 400px" /div> https://vizhub.healthdata.org/subnational/usa --- <div style="margin-top:-30px"></div> # Model <!-- PM2.5 concentration in los Angeles and Ventura counties, 2011 `\(Y(\boldsymbol{x})\)` air pollution measurement (PM2.5) at location `\(\boldsymbol{x} \in \mathbb{R}^2\)`<br> --> Assume there is a spatially continuous variable underlying all observations that can be modeled using a Gaussian random field `\(S=\{S(\boldsymbol{x}): \boldsymbol{x} \in D \subset \mathbb{R}^2 \}\)`, `\(E[S(\boldsymbol{x})]=0\)`, `\(Cov(S(\boldsymbol{x}), S(\boldsymbol{x}')) = \Sigma(\boldsymbol{x}-\boldsymbol{x}')\)` Model `$$Y(\boldsymbol{x})|S(\boldsymbol{x}) \sim N(\mu(\boldsymbol{x})+S(\boldsymbol{x}),\tau^2)$$` - Point data observed at `\(\boldsymbol{x}_i \in D\)` `$$E[Y(\boldsymbol{x}_i)] = \mu(\boldsymbol{x}_i)+S(\boldsymbol{x}_i)$$` - Areal data arise as block averages in blocks `\(B_j \subset D\)` `$$E[Y(B_j)]=|B_j|^{-1}\int_{B_j}(\mu(\boldsymbol{x})+S(\boldsymbol{x}))d\boldsymbol{x},\ |B_j|>0$$` --- <div style="margin-top:-30px"></div> # Inference using INLA and SPDE Integrated nested Laplace approximations (INLA) is an approach to perform approximate Bayesian inference in latent Gaussian models - Observed data `\(\boldsymbol{y}\)`: `\(y_i| \boldsymbol{x}, \boldsymbol{\theta} \sim \pi(y_i | x_i, \boldsymbol{\theta})\)` - Latent Gaussian field: `\(\boldsymbol{x}|\boldsymbol{\theta} \sim N(\boldsymbol{\mu(\theta)}, \boldsymbol{Q(\theta)^{-1}})\)` - Hyperparameters: `\(\theta \sim \pi(\boldsymbol{\theta})\)` INLA provides a huge improvement of speed compared to MCMC alternatives - Laplace approximations to obtain posterior marginals - Numerical algorithms for sparse matrices The combination of INLA and Stochastic Partial Differential Equations (SPDE) approaches permits to analyze point-level data INLA and SPDE can be applied using the R package `R-INLA` <!-- #--- # Air pollution prediction Predict PM2.5 concentration in los Angeles and Ventura counties, 2011. <center> <div style="margin-top:10px; width:100%;"> <img src="./figures/realpointdatapollution2.png" style="width:45%;"/> <img src="./figures/realpointandarealdatapollution2.png" style="width:45%;"/> </div> </center> Point data observed at `\(\boldsymbol{x}_i \in D\)` `$$E[Y(\boldsymbol{x}_i)] = \mu(\boldsymbol{x}_i)+S(\boldsymbol{x}_i)$$` Areal data arise as block averages in blocks `\(B_j \subset D\)` `$$E[Y(B_j)]=|B_j|^{-1}\int_{B_j}(\mu(\boldsymbol{x})+S(\boldsymbol{x}))d\boldsymbol{x},\ |B_j|>0$$` --> --- <div style="margin-top:-40px"></div> # Inference using INLA and SPDE <div style="margin-top:-10px"></div> In the SPDE approach, the continuously indexed Gaussian field S is represented as a discretely indexed Gaussian Markov random field (GMRF) by means of a finite basis function defined on a triangulation of the study region `$$S(\boldsymbol{x}) = \sum_{g=1}^G \psi_g(\boldsymbol{x}) S_g$$` - `\(\psi_g(\cdot)\)` piecewise polynomial basis functions on each triangle - `\(\{S_g \}\)` zero-mean Gaussian distributed - `\(G\)` number of vertices in the triangulation <center> <img src="./figures/pointsandtriang.png" style="width:38%;"/> </center> --- # Point observations `\(S(\boldsymbol{x})\)` weighted average of the GMRF values at the vertices of the triangle containing the point. Weights = barycentric coordinates `$$S(\boldsymbol{x}) \approx \frac{T_{1}}{T}S_1 + \frac{T_{2}}{T}S_2 + \frac{T_{3}}{T}S_3$$` `\(T_1, T_2, T_3\)` areas subtriangles formed by `\(\boldsymbol{x}\)` and vertices. `\(T\)` area whole triangle <center> <img src="./figures/triangleweights.png" style="width:40%;"/> </center> <!-- Code to create image triangleweights.png C:\presentations\presentation-misaligned-air-pollution --> --- # Areal observations `\(S(B)=|B|^{-1} \int_{B} S(\boldsymbol{x})d\boldsymbol{x}\)` weighted average of the GMRF values at the vertices of the triangles within the area. Weights = `\(\mbox{ (number vertices)}^{-1}\)` `$$S(B) \approx \frac{1}{m} \sum_{g \in B} S_g$$` <center> <img src="./figures/pointsinareaandtriang.png" style="width:40%;"/> </center> --- # Projection matrix `$$S(\boldsymbol{x}_i) \approx \sum_{g=1}^G A_{ig} S_g\ \ \ \ \ \ \ \ \ \ \ S(B_j) \approx \sum_{g=1}^G A_{jg} S_g$$` where `\(A\)` is a projection matrix that maps the GMRF from the observations to the triangulation nodes. - Row `\(i\)` in `\(A\)` of point observation: possibly three non-zero values at columns that represent the vertices of the triangle containing the point (= barycentric coordinates). - Row `\(j\)` in `\(A\)` of areal observation: non-zero values in all the m vertices inside the area (= `\(1/m\)`). `$$A = \begin{bmatrix} A_{11} & A_{12} & A_{13} & \dots & A_{1G} \\ A_{21} & A_{22} & A_{23} & \dots & A_{2G} \\ \vdots & \vdots & \vdots & \ddots & \vdots \\ A_{n1} & A_{n2} & A_{n3} & \dots & A_{nG} \end{bmatrix} = \begin{bmatrix} 0 & 0 & 1 & \dots & 0 \\ .2 & .2 & 0 & \dots & .6 \\ \vdots & \vdots & \vdots & \ddots & \vdots \\ 1/m & 1/m & 1/m & \dots & 0 \end{bmatrix}$$` --- # Malaria prevalence in Madagascar, 2012 Predicting malaria prevalence by combining point and areal data `$$\begin{aligned} Y(\mathbf{x}) & \sim \operatorname{Binomial}\left(N(\mathbf{x}), P(\mathbf{x})\right), \quad \mathbf{x} \in A \subset \mathbb{R}^2, \\ P(\mathbf{x_i}) & = \text{logit}^{-1}\left(\mu(\mathbf{x}_i)+S(\mathbf{x}_i)\right), \quad i=1, \ldots, n, \\ P(B_i) & = \left|B_i\right|^{-1} \int_{B_i} \text{logit}^{-1} \left(\mu (\mathbf{x}) + S(\mathbf{x}) \right) d \mathbf{x}, \quad i=n+1, \ldots, n+m. \end{aligned}$$` <center> <img src="./figures/misaligned-malaria-pointdata.png" style="width:30%;margin-left:-10%;"/> <img src="./figures/misaligned-malaria-arealdata.png" style="width:30%;margin-left:-10%;"/> <img src="./figures/misaligned-malaria-altitude.png" style="width:30%;margin-left:-10%;"/> <img src="./figures/misaligned-malaria-excprob.png" style="width:30%;margin-left:-10%;"/> </center> <!-- <img src="./figures/misaligned-malaria-predmean.png" style="width:20%;"/> --> <!-- [Alahmadi and Moraga, JRSSA, 2024](https://doi.org/10.1007/s13253-023-00559-w) --> --- # Bayesian spatial disaggregation modelling for the detection of disease clusters Exceedance probabilities derived from Bayesian spatial models can be used to detect high-risk areas. However, clusters can have shapes different from areas. For example: <center> <img src="./figures/clusters-shapes.png" style="width:40%;"/> </center> We propose to detect clusters by first obtaining risk surfaces from a Bayesian spatial disaggretation model, and then use exceedance probabilities to identify high-risk locations `$$P(\theta(\mathbf{x}) > \mbox{threshold})$$` This method shows high sensitivity and specifity in a range of scenarios in comparison with other existing clustering methods. --- class: inverse, center, middle # Spatio-temporal modeling of infectious diseases by integrating compartment and point process models --- <div style="margin-top:-20px"></div> # Spatio-temporal prediction of COVID-19 in Cali, Colombia, by integrating compartment and point process models <img src="./figures/anim.gif" style="width:49%;"/> <img src="./figures/animtime.gif" style="width:49%;"/> [Ribeiro Amaral, et al. SERRA, 2022](https://doi.org/10.1007/s00477-022-02354-4) --- <div style="margin-top:-20px"></div> # SIR + LGCP model Two-step approach: Aggregate data in the whole region for each time. Fit temporal SIR (Susceptible, Infected, Recovered) compartment model to obtain the predicted number of infectious individuals at each time `$$\begin{align*} \frac{dS(t)}{dt} &= -\beta S(t) \frac{I(t)}{N} \\ \frac{dI(t)}{dt} &= +\beta S(t) \frac{I(t)}{N} - \gamma I(t) \\ \frac{dR(t)}{dt} &= +\gamma I(t) \end{align*}$$` Number of individuals in the population `\(S(t) + I(t) + R(t) = N\)` constant Disease parameters: `\(\beta > 0\)` infectious rate, `\(\gamma > 0\)` recovery or death rate We extend SIR model to allow for the incorporation of age-stratified contact information, which provides valuable information for decision-making SIR model could be extended to consider more compartments (e.g.,<br> `\(I_S(t)\)` symptomatic, `\(I_A(t)\)` asymptomatic) --- <div style="margin-top:0px"></div> # SIR + LGCP model Fit a log-Gaussian Cox process for the locations of infected individuals in the studied region and time, with mean depending on the predicted number of infected people at that time obtained from the SIR model <br> `$$\begin{align} N(A, t) &\sim \text{Poisson}\left(\int_{A}\Lambda(\mathbf{x}, t)d\mathbf{x}\right) \\ \Lambda(\mathbf{x}, t) &= \lambda_{0}(\mathbf{x}, t)\ I(t)\ \exp(S({\mathbf{x}, t})) \nonumber \\ \end{align}$$` `$$\begin{align} \lambda_{0}(\mathbf{x}, t):\ &\text{Proportional to the population density and integrates to 1}\\ I(t):\ &\text{Number of infected people at time } t\ \text{obtained from SIR model}\\ S(\mathbf{x}, t):\ &\text{Spatial Gaussian random field with Matern covariance function} \nonumber \\ \end{align}$$` --- # COVID-19 prediction in Cali, Colombia <img src="./figures/covid19cali2.png" style="width:100%;"/> 4518 COVID-19 locations from 21/1/2020 to 18/6/2020 <!-- `\(\text{AAPE}_{i, j, k} = \arctan\left(\left|{(f_{i, j, k} - \hat{f}_{i, j, k})}/{f_{i, j, k}}\right|\right)\)` --> Using simulations and real COVID-19 data, we showed SIR+LGCP model has better forecasting performance than other LGCP models that do not do use information from the SIR model SIR+LGCP model can help decision-makers to identify high-risk locations and vulnerable populations to develop better strategies for infectious diseases prevention and control --- class: inverse <br> # Spatial epidemiology studies <br> ## Lymphatic filariasis in sub-Saharan Africa ## Leptospirosis in Brazil ## Mortality risk attributable to temperature in India ## Effects of temperature on mental health in Spain <!-- #--- class: inverse, center, middle # Lymphatic filariasis in sub-Saharan Africa ### Modelling the distribution and transmission intensity of lymphatic filariasis in sub-Saharan Africa prior to scaling up interventions: integrated use of geostatistical and mathematical modelling ### [Moraga, et al., Parasites & Vectors, 8:560, 2015](https://doi.org/10.1186/s13071-015-1166-x) --> --- <div style="margin-top:-35px"></div> # Geospatial modelling of lymphatic filariasis prevalence in sub-Saharan Africa <div style="margin-top:-35px"></div> .pull-left-60[ Lymphatic filariasis (LF) caused by microscopic worms and transmitted by mosquitoes Disfigurement and disabilities due to lymphedema, elephantiasis and hydrocele <img src="./figures/lflifecycle.JPG" style="width:90%;"/> ] .pull-right-40[ <img src="./figures/lfAedesaegypti.jpg" style="width:80%;"/> <img src="./figures/lfsymptoms1.jpg" style="width:80%;"/> ] --- background-image: url(./figures/lfburdenourworldindata1.png) background-size: contain <!-- https://ourworldindata.org/grapher/prevalence-of-lymphatic-filariasis) --> <div style="margin-top:600px"></div> Main strategy against the disease is Mass Drug Administration.<br> Resources are limited and need to decide which are the areas most in need. <!-- #--- <div style="margin-top:-20px"></div> # Geospatial modelling of lymphatic filariasis prevalence in sub-Saharan Africa Main strategy against the disease is **Mass Drug Administration** (recommended to entire populations in regions where prevalence exceeds 1% annually for at least five years) Resources are limited and need to decide which are the areas most in need <img src="./figures/mda1.png" style="width:46%;"/> <img src="./figures/lfChildtreatmentfilariasis.JPG" style="padding-bottom:0px; width:50%;"/> --> <!-- Geographical targeting of interventions is required to ensure programmes are implemented appropriately --> <!-- https://cntd.lstmed.ac.uk/our-work/themes/mass-drug-administration-mda https://ourworldindata.org/grapher/prevalence-of-lymphatic-filariasis https://apps.who.int/iris/bitstream/handle/10665/250245/WER9139.pdf;jsessionid=BFDC2323CD77FB87F3856DF837A3B468?sequence=1 https://ig.ft.com/special-reports/elephantiasis/ --> <!-- #--- <div style="margin-top:-10px"></div> # LF prevalence surveys in sub-Saharan Africa <center> <img src="./figures/africaICTr.png" style="margin-left:-40px; width:75%;"/> </center> --> <!-- <div style="width:20%;"> 3197 surveys 1990-2014 </div> <div style="width:30%; margin-left:-50px; margin-bottom:-180px"> <img src="./figures/lficttest.jpg" style="width:100%;"/> </div> <center> <img src="./plots/africaICT.png" style="margin-left:10px; width:55%;"/> </center> --> --- <div style="margin-top:-30px"></div> # Geospatial modelling of lymphatic filariasis prevalence in sub-Saharan Africa <center> <div style="margin-left:-80px; margin-right:-20px; width:120%;"> <img src="./figures/mbg.png" style="width:100%;"/> </div> </center> <div style="margin-top:-30px"></div> 3197 LF prevalence surveys, 1990-2014 --- <div style="margin-top: -35px;"> # Predict local LF prevalence <div style="margin-top: -10px;"> `$$Y_i|P(\boldsymbol{x}_i)\sim \mbox{Binomial} (n_i, P(\boldsymbol{x}_i))$$` `$$\mbox{logit}(P(\boldsymbol{x}_i)) = \boldsymbol{z}_i \boldsymbol{\beta} + S(\boldsymbol{x}_i) + u_i$$` Covariates based on characteristics known to affect LF transmission (precipitation, vegetation, elevation, land cover, population density, etc.) <div style="margin-top: -10px;"> <!-- (temperature, precipitation, vegetation, elevation, distance to water bodies, urbanization, land cover, population density, etc) Random effects (Gaussian process with Matern covariate funcion, Independent risk) --> Random effects model residual variation not explained by covariates <center> <img src="./figures/covgrf.png" style="width:97%;"/> </center> --- background-size: contain .pull-left-50[ # LF predictions ### Maps can help surveillance activities by ### - identifying areas that require enhanced interventions ### - setting a benchmark for evaluating the impact of interventions ] .pull-right-50[ <br><br><br> 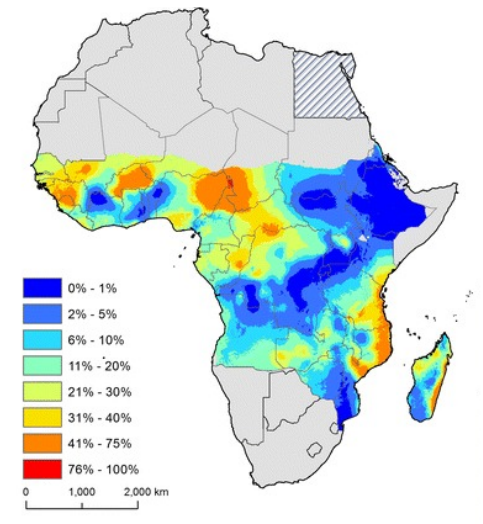 ] [Moraga, et al., Parasites & Vectors, 2015](https://doi.org/10.1186/s13071-015-1166-x) <!-- #--- class: inverse, center, middle # Leptospirosis in Brazil ### Spatio-temporal determinants of urban leptospirosis transmission: Four-year prospective cohort study of slum residents in Brazil ### [Hagan, Moraga, et al., PLOS Neglected Tropical Diseases,<br> 10(1): e0004275, 2016](https://journals.plos.org/plosntds/article?id=10.1371/journal.pntd.0004275) --> --- <div style="margin-top:-30px"></div> # Spatio-temporal determinants of urban leptospirosis transmission in Brazil <div style="margin-top:-15px"></div> <!-- # Spatio-temporal determinants of urban leptospirosis transmission: Four-year prospective cohort study of slum residents in Brazil --> .pull-left-50[ **Transmission** via direct skin or mucosal contact with animal reservoirs or an environment contaminated with infected urine **Manifestations**: fever, headache, myalgia, aseptic meningitis, jaundice, renal failure, pulmonary hemorrhage syndrome Urban health problem due to the **rapid and disorganized expansion of urban centers**, creating ecological conditions for rat-borne transmission Predominantly affects **vulnerable populations** such as subsistence farmers and residents of urban<br> slums in the tropics ] <!-- https://www.google.com/search?safe=strict&rlz=1C1GCEV_en&biw=1920&bih=969&tbm=isch&sxsrf=ACYBGNSCjyvzOzkeqzz5w6K8zXqMVDhAKA%3A1575918696756&sa=1&ei=aJzuXZPeLZu6gAaiiLPwBA&q=leptospirosis+cycle&oq=leptospirosis+cycle&gs_l=img.3..0j0i8i30l5.65261.65982..66461...0.0..0.210.916.5j2j1......0....1..gws-wiz-img.......35i39j0i67j0i7i30j0i24j0i5i30.hI2NxAn9BJw&ved=0ahUKEwiTsvXQoqnmAhUbHcAKHSLEDE4Q4dUDCAc&uact=5#imgrc=b2D4YGJZcyKHeM: --> .pull-right-50[ <img src="./figures/cyclelepto.jpg" style="margin-top:10px; padding-left:20px; width:90%;"/> ] --- # Leptospirosis in Pau da Lima, Brazil <!-- Leptospirosis emerging as a major urban health problem due to the rapid and disorganized expansion of urban centers, creating ecological conditions for rat-borne transmission Pau da Lima is a urban slum in Salvador, Bahia, that hosts a densely populated community with a high annual incidence of leptospirosis --> Pau da Lima is a urban slum in Salvador, Bahia, that hosts a densely populated community with a high annual incidence of leptospirosis <center> <div style="margin-left:-80px; margin-right:-20px; width:122%;"> <img src="./figures/salvador.jpg" style="width:42%;"/> <img src="./figures/paudalimaaerial1.jpg" style="width:57.2%;"/> </div> </center> --- <center> <div style="margin-top:-20px; margin-left:-80px; margin-right:-20px; width:122%;"> <img src="./figures/paudalimaelevationj.jpg" style="width:50%;"/> <img src="./figures/paudalimaropaj.jpg" style="width:49%;"/> <img src="./figures/paudalimaclearingoutopensewer.jpg" style="width:50%;"/> <img src="./figures/paudalimagarbagecollectionj.jpg" style="width:49%;"/> </div> </center> --- <div style="margin-top: -20px;"> # Leptospirosis in Pau da Lima <div style="margin-top: -10px;"> **Objective**: Understanding the spatio-temporal patterns of leptospirosis and identifying targets for intervention in Pau da Lima **Data**: 2003 subjects recruited and followed for a four-year period (2003-2007). Annual administration of questionnaires and GIS mapping to determine socio-behavioral and environmental risk factors. ### Spatio-temporal mixed model <div style="margin-top: -10px;"> `$$Y_{ij}| P(\boldsymbol{s}_i, j) \sim Bernoulli(P(\boldsymbol{s}_i, j))$$` `$$logit(P(\boldsymbol{s}_i, j))= \boldsymbol{z}_{ij} \boldsymbol{\beta} + \xi(\boldsymbol{s}_i,j) + u_i$$` `$$\xi(\boldsymbol{s}_i,j)=a \xi(\boldsymbol{s}_i,j-1)+w(\boldsymbol{s}_i,j)$$` `$$|a|<1, \xi(\boldsymbol{s}_i,1) \sim N(0, \sigma_w^2/ (1-a^2)), w(\boldsymbol{s}_i,j) \sim N(0,\sigma^2_w C(h))$$` `$$u_i \sim N(0, 1/\tau_u)$$` <br> [Hagan, et al., PLOS Neglected Tropical Diseases, 2016](https://journals.plos.org/plosntds/article?id=10.1371/journal.pntd.0004275) --- background-image: url(./figures/variables.png) background-size: contain <!-- #--- # Selection fixed effects Within each group, fit GAM using all categorical variables, non-linear effects of continuous variables, and interactions between them Remove covariates one by one until obtaining a model with minimum AIC - Demographic and social status factors `\(\longrightarrow\)` V1 - Health factors `\(\longrightarrow\)` V2 - Occupational exposures `\(\longrightarrow\)` V3 - Household environment `\(\longrightarrow\)` V4 - Household behaviour `\(\longrightarrow\)` V5 - Household reservoirs `\(\longrightarrow\)` V6 - Work behaviour `\(\longrightarrow\)` V7 - Work reservoirs `\(\longrightarrow\)` V8 Merge groups and explore further simplification by backward elimination until it is no longer possible to reduce AIC V1+V2+V3+V4+V5+V6+V7+V8 `\(\longrightarrow\)` V --> <!-- #--- # Selection fixed effects Model includes: - year of follow-up (1st, 2nd, 3rd or 4th year) - gender (male/ female) - being literate (literate / illiterate) - house elevation - observation of rats near house (observe/ not observe) - contact with mud near house (contact / no contact) - non-linear effect of age <center> <img src="./figures/gamagefinalmodelt.png" style="margin-top:-40px; margin-left:180px; width:46%;"/> </center> --> --- # Risk factors <div style="margin-top: -20px;"> <img src="./figures/riskfactors.png" style="width:95%;"/> </div> <!-- #--- # Areas with highest (red) and lowest (blue) odds of infection <center> <div style="margin-top: -10px; margin-left:-50px; margin-right:-50px; width:75%;"> <img src="./figures/highestlowestodds2.png"/> </div> </center> --> <style> .contentlepto { width: 50%; position: relative; /* Position the background text */ left: 0; /* top: 0; left: 0; right: 0; At the bottom. Use top:0 to append it to the top */ background: rgb(0, 0, 0); /* Fallback color */ background: rgba(0, 0, 0, 0.5); /* Black background with 0.5 opacity */ color: #f1f1f1; /* Grey text */ width: 100%; /* Full width */ padding: 10px; /* Some padding */ } </style> --- <div style="margin-top:-20px;"> <h1>High-risk region</h1> <p style="margin-top:-20px; margin-bottom:20px;">High-risk region in which measured risk is consistent with prediction <p> </div> <center> <div style="margin-left:-20px; width:100%;"> <img style="margin-left:-20px; width:90%;" src="./figures/highriskr.png"/> </div> </center> --- <div style="margin-top:-20px;"> <h1>High positive residual</h1> <p style="margin-top:-20px; margin-bottom:20px;">Poor household construction and flood risk due to poor infrastructure <p> </div> <center> <div style="width:52%;"> <img src="./figures/positiveresidual.png"/> </div> </center> --- <div style="margin-top:-20px;"> <h1>High negative residual</h1> <p style="margin-top:-20px; margin-bottom:20px;">Open sewers with structural barriers to stop overflow <p> </div> <center> <div style="margin-left:-80px; margin-right:-20px; width:125%;"> <img src="./figures/negativeresidual1.png" style="width:35.8%;"/> <img src="./figures/negativeresidual2.png" style="width:63.5%;"/> </div> </center> <style> .pull-left-70 { float: left; width: 70%; } .pull-right-30 { float: right; width: 30%; } </style> <!-- #--- # Conclusions <br> - Young adults, males, and individuals with low social status may have activities that place them in more frequent or more intense contact with sources of transmission <div style="margin-top:40px;"></div> - Interventions such providing adequate sanitation systems may reduce the burden of leptospirosis by protecting residents from contact with contaminated soil and mud during heavy rain events <div style="margin-top:40px;"></div> - Areas in which transmission risk cannot be adequately explained using known risk factors enable the identification of new hypotheses about transmission and guide targeted interventions --> --- class: inverse, center, middle # Statistical software --- background-image: url(./figures/overviewsoftware2.png) background-size: contain # Statistical software <div style="color: gray; height: 20px;bottom:100px;left: 80px;position: fixed;"> <br><br> <a href='https://f1000research.com/articles/7-1374/v3' target='_blank'>Moraga, et al. F1000 Research, 7:1374, 2019</a><br> <a href='https://doi.org/10.1016/j.sste.2017.08.001' target='_blank'>Moraga. Spatial and Spatio-temporal Epidemiology, 23:47-57, 2017</a><br> </div> --- <div style="margin-top:-40px"></div> # SpatialEpiApp <div style="margin-top:-10px"></div> Shiny app for disease risk estimation, cluster detection, and interactive viz - Risk estimates by fitting Bayesian models with [INLA](http://www.r-inla.org/) - Detection of clusters by using the scan statistics in [SaTScan](https://www.satscan.org/) http://www.paulamoraga.com/software/ ```r library(devtools) install_github("Paula-Moraga/SpatialEpiApp") library(SpatialEpiApp) run_app() ``` <img src="./figures/animation.gif" width="100%" style="display: block; margin: auto;" /> --- <div style="margin-top:-30px"></div> <b>Upload map and data<b> <div style="margin-top:-5px"></div> <img src="./figures/exinput.png" style="width:96%;"/> <div style="margin-top:-10px"></div> <b>Bayesian disease risk models Scan statistics for detection of clusters<b> <div style="margin-top:-5px"></div> <img src="./figures/exmaps.png" style="width:44%;"/> <img src="./figures/exclusters.png" style="width:52%;"/> --- # epiflows. Travel-related spread of disease <div style="color: gray; height: 30px;bottom:10px;left: 80px;position: fixed;"> <a href='https://f1000research.com/articles/7-1374/v3' target='_blank'>Moraga, et al. F1000 Research, 7:1374, 2019</a><br> </div> <img src="./figures/epiflows2.png" style="width:57%;"/> <img src="./figures/epiflows.gif" style="width:42%;"/> </center> Mathematical model that predicts the number of cases that could be spread to other locations from an infectious location together with uncertainty measures Integrates information about the number of infectious cases, population flows, lengths of stay, incubation and infectious periods --- <div style="margin-top:-20px"></div> **Total infections introduced in O from S = Exportations + Importations** **Exportations** `$$E_{S,O}= C_{S,W} \times p_O \times p_i$$` - `\(C_{S,W}\)` cumulative number of infections in location `\(S\)` and time window `\(W\)` - `\(p_O = \frac{T_{S,O}^W}{pop_S}\)` per capita probability that a resident of `\(S\)` travels to `\(O\)` - `\(T_{S,O}^W\)` residents of `\(S\)` travelling to `\(O\)`. `\(pop_S\)` population `\(S\)` - `\(p_i = min\left(\frac{D_E+D_I}{W}, 1\right)\)` prob. infection incubated or infectious during `\(W\)` **Importations** `$$I_{S,O} = T_{O,S}^W \times \lambda_S \times p_l$$` - `\(T_{O,S}^W\)` number travelers visiting `\(S\)` from `\(O\)` in time window `\(W\)` - `\(\lambda_S = \frac{C_{S,W}\times L_0}{pop_S \times W}\)` per capita risk infection of travelers visiting `\(S\)` during stay - `\(L_0\)` average length of stay - `\(p_l = min\left(\frac{D_E+D_I}{L_0}, 1\right)\)` prob. return home while incubating or infectious **Uncertainty** obtained by sampling from incubation `\(D_E\)` and infectious `\(D_I\)` period distributions. This produces a full distribution for `\(p_i\)` and `\(p_l\)`, and in turn to exportations, importations, and total number of infections <!-- #--- background-image: url(./figures/recon.png) background-size: contain <div style="color: gray; height: 20px;bottom:70px;left: 80px;position: fixed;"> <br><br> <a href='https://www.repidemicsconsortium.org/' target='_blank'>https://www.repidemicsconsortium.org/</a><br> </div> #--- <img src="./figures/afrimapr.png" style="margin-top:-30px; margin-bottom:-20px; margin-left:30px; width:95%;"/> https://afrimapr.github.io/afrimapr.website/ --> --- class: inverse # Current Research <div style="margin-top:80px;"> ## Statistical methods and computational tools to solve challenging problems and improve population health <div style="margin-top:40px;"> ## 1. Digital health surveillance ## 2. Interactive visualization apps --- class: inverse, center, middle # 1. Digital health surveillance --- # Disease surveillance systems Disease surveillance systems are critical to early detection of epidemics and the design of control strategies Traditional surveillance systems rely on data gathered with a considerable delay and make surveillance systems ineffective for real-time surveillance <br> <!-- Public - Clinicians - Local Health Departments - Ministries of Health --> <center> <div style="width:100%;"> <img src="./figures/cold-cover-handkerchief-41284.jpg" style="margin-left:-20px; width:14%;"/> <img src="./figures/arms-care-check-905874.jpg" style="width:35%;"/> <img src="./figures/biology-clinic-doctor-4154.jpg" style="width:31%;"/> <img src="./figures/healthdepartments.png" style="width:18%;"/> </div> --- # Digital data sources Real-time digital information may enable to detect outbreaks earlier <center> <img src="./figures/apple-applications-apps-607812.jpg" style="width:70%;"/> <br> <span style="color: gray">"Flu plus fever, not a good way to start the weekend"</span> <br> <span style="color: gray">"I'm so irritated at this cough and fever"</span> <br> <span style="color: gray">"This flu, fever & throat ache won't let me sleep"</span> </center> --- # Demographic and environmental risk factors <img src="./figures/eo.gif" style="width:100%;"/> <!-- ## Existing approaches do not produce inferences at spatial resolutions actionable by policymakers <center> <img src="./images/gft.png" style="width:90%;"/> <img src="./images/ARGO.png" style="width:60%;"/> <img src="./images/healthmap.png" style="width:30%;"/> </center> [The New York Times, 2008](https://www.nytimes.com/), [Yang et al., 2015, PNAS](https://doi.org/10.1073/pnas.1515373112), [HealthMap, 2018, PNAS](http://www.healthmap.org/en/) --> <!-- - Policy making need data at a spatial scale that is actionable - However, existing methods produce inferences at regional or national level - Healthmap provide local information but not inferences --> --- <div style="margin-top:-30px"></div> # Digital health surveillance system <div style="margin-top:-10px"></div> - **Data-gathering platform** - **Modelling framework** that integrates multiple data sources so as to produce local probabilistic predictions of disease activity in real-time - **Interactive dashboard** that alert public health officials when elevated disease levels are anticipated, and provide insights about disease drivers <center> <img src="./figures/datadigital2.png" style="width:80%;"/> </center> <!-- - display of current and historical disease patterns - disease predictions - notifications of outbreaks - reports summarizing disease level - overlay of maps with contextual information # Disease surveillance system into practice - System portable and accessible by health departments worldwide - Put the surveillance system into practice by collaborating with national and international partners - This information will be used to respond to public health threats in a timely and appropriate manner Integrates the data-gathering platform, the modelling architecture and a dashboard including interactive data visualizations and reports --> --- <div style="margin-top: -20px;"> # Innovation in data acquisition and analysis <img src="./figures/SDGsDataStreams.png" style="width:95%;"/> </div> [Fritz et al. Nature Sustainability (2019)](https://doi.org/10.1038/s41893-019-0390-3) [Moraga and Baker. F1000 Research (2022)](https://f1000research.com/articles/11-770/v1) --- class: inverse, center, middle # 2. Development of interactive visualization applications for policymaking --- <div style="margin-top:-10px"></div> # Interactive visualizations for policymaking <div style="color: gray; height: 30px;bottom:10px;left: 80px;position: fixed;"> <a href='https://doi.org/10.1016/j.sste.2017.08.001' target='_blank'>Moraga. Spatial and Spatio-temporal Epidemiology, 23:47-57, 2017</a><br> </div> Effective communication and proper and timely dissemination of information for the development and implementation of population health policies - Digital web health atlases (cancer, cardiovascular, infectious diseases) - Digital health surveillance systems to monitor diseases in real-time <div style="margin-top:20px"></div> 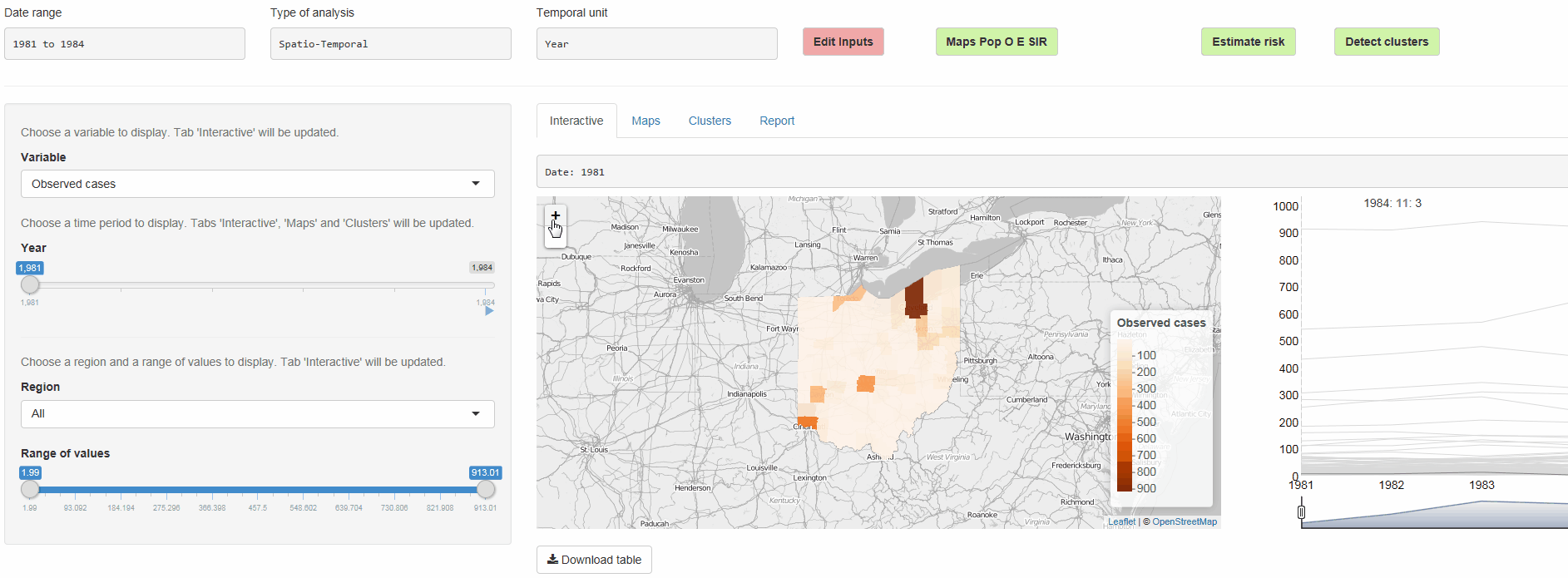 <a href="https://paulamoraga.shinyapps.io/spatialepiapp/"> https://paulamoraga.shinyapps.io/spatialepiapp/</a> <!-- #--- background-image: url(./figures/kaustpositions-fellowship.png) background-size: contain --> --- background-image: url(./figures/Admissions-com-2020-COVID.png) background-size: contain background-position: top right <div style="margin-top:180px"></div> # Join my research group at KAUST! <div style="margin-top:-10px"></div> **KAUST** is an international research university located on the shores of the Red Sea in Saudi Arabia. KAUST is dedicated to advancing science and technology through interdisciplinary research, education and innovation. **KAUST Fellowship**. All admitted students receive free tuition, annual living allowance, free housing, medical and dental coverage, relocation support <!-- Research areas of global significance: Food & Health, Water, Energy, Environment, Digital --> <div style="margin-top:20px"></div> ### KAUST http://kaust.edu.sa ### Statistics Program http://stat.kaust.edu.sa ### Admissions http://admissions.kaust.edu.sa <!-- http://studyat.kaust.edu.sa --> --- background-image: url(./figures/Admissions-com-2020-COVID.png) background-size: contain background-position: top right <div style="margin-top:180px"></div> # Join my research group at KAUST! <div style="margin-top:-20px"></div> I am looking for outstanding PhD students and Postdocs to join my group <div style="margin-top:-10px"></div> 👩💻 Potential research areas include the development of innovative statistical and computational methods for health and environmental applications - Disease mapping, cluster detection, early detection of outbreaks - Integration of spatial and spatio-temporal data - Development of R packages for data analysis and visualization 💪 Work closely with collaborators at KAUST and around the world ✈️ Generous travel funding for conferences and collaborative work ✨ Excellent research environment. Superb equipment and research facilities <!-- **KAUST Fellowship**. All admitted students receive free tuition, annual living allowance, free housing, medical and dental coverage, relocation support --> <a href='http://twitter.com/Paula_Moraga_' target='_blank'> <i class='fa fa-twitter fa-fw'></i> @Paula\_Moraga_</a> <a href='https://Paula-Moraga.github.io/' target='_blank'> <i class='fa fa-globe fa-fw'></i> www.PaulaMoraga.com</a> <a href='https://www.kaust.edu.sa/en' target='_blank'> <i class='fa fa-graduation-cap fa-fw'></i> www.kaust.edu.sa</a><br> <!-- https://emoji.muan.co/#rais --> --- <div style="margin-top:-30px;"></div> <!-- Moraga, P. (2019). *Geospatial Health Data: Modeling and Visualization with R-INLA and Shiny*. Chapman & Hall/CRC Press --> # References <small> <div style="margin-top:-30px;"></div> .pull-left-70[ Ribeiro Amaral, A., et al. (2022). Spatio-temporal modeling of infectious diseases by integrating compartment and point process models. *SERRA*, 37, 1519-1533 Moraga, P., et al. (2019). epiflows: an R package for risk assessment of travel-related spread of disease. *F1000Research*, 7:1374 Moraga, P. (2018). Small Area Disease Risk Estimation and Visualization Using R. *The R Journal*, 10(1):495-506 Moraga, P. (2017). SpatialEpiApp: A Shiny Web Application for the analysis of Spatial and Spatio-Temporal Disease Data. *Spatial and Spatio-temporal Epidemiology*, 23:47-57 Moraga, P., et al. (2017). A geostatistical model for combined analysis of point-level and area-level data using INLA and SPDE. *Spatial Statistics*, 21:27-41 <!-- Moraga, P. and Kulldorff, M. (2016). Detection of spatial variations in temporal trends with a quadratic function. *Statistical Methods for Medical Research*, 25(4):1422-1437 --> Hagan, J. E., Moraga, P., et al. (2016). Spatio-temporal determinants of urban leptospirosis transmission: Four-year prospective cohort study of slum residents in Brazil. *PLOS Neglected Tropical Diseases*, 10(1): e0004275 Moraga, P., et al. (2015). Modelling the distribution and transmission intensity of lymphatic filariasis in sub-Saharan Africa prior to scaling up interventions: integrated use of geostatistical and mathematical modelling. *Parasites & Vectors*, 8:560 ] .pull-right-30[ <img src="./figures/bookcoverspatial.jpg" style="width:80%; padding-left:30px;"/> <br> <img src="./figures/bookcover.jpg" style="width:80%; padding-left:30px;"/> ] <div style="margin-bottom:-40px;"> </div> </small> --- class: inverse <table style="margin:0px; margin-left:-10px; border-top:0; border-bottom:0;"> <tr> <td style="width: 440px;"> <div style = 'margin-top: 60px; margin-bottom: 40px;'> <span style = 'font-size: 68px; line-height:1.5; font-weight:bold'> Thanks!<br> </span> </div> <span style = 'font-size: 38px; font-weight:bold'> Paula Moraga<br> </span> <br> <span style = 'font-size: 28px; line-height:1.5'> <a href='http://twitter.com/Paula_Moraga_' target='_blank'> <i class='fa fa-twitter fa-fw'></i> @Paula_Moraga_</a><br> <a href='https://Paula-Moraga.github.io/' target='_blank'><i class='fa fa-globe fa-fw'></i> www.PaulaMoraga.com</a><br> </span> </td> <td> <div style = 'margin-top: 120px; margin-bottom: 40px;'> </div> <center> <img src="./figures/logogeohealthdarkbackground.png" height = "220" alt = "a png"><br><br> <img src="./figures/Statistics at KAUST_Logo for digital use_small.png" height = "160" alt = "a png"> </center> </td> </tr> </table> </div>